Transforming Business with Data Labeling in Machine Learning
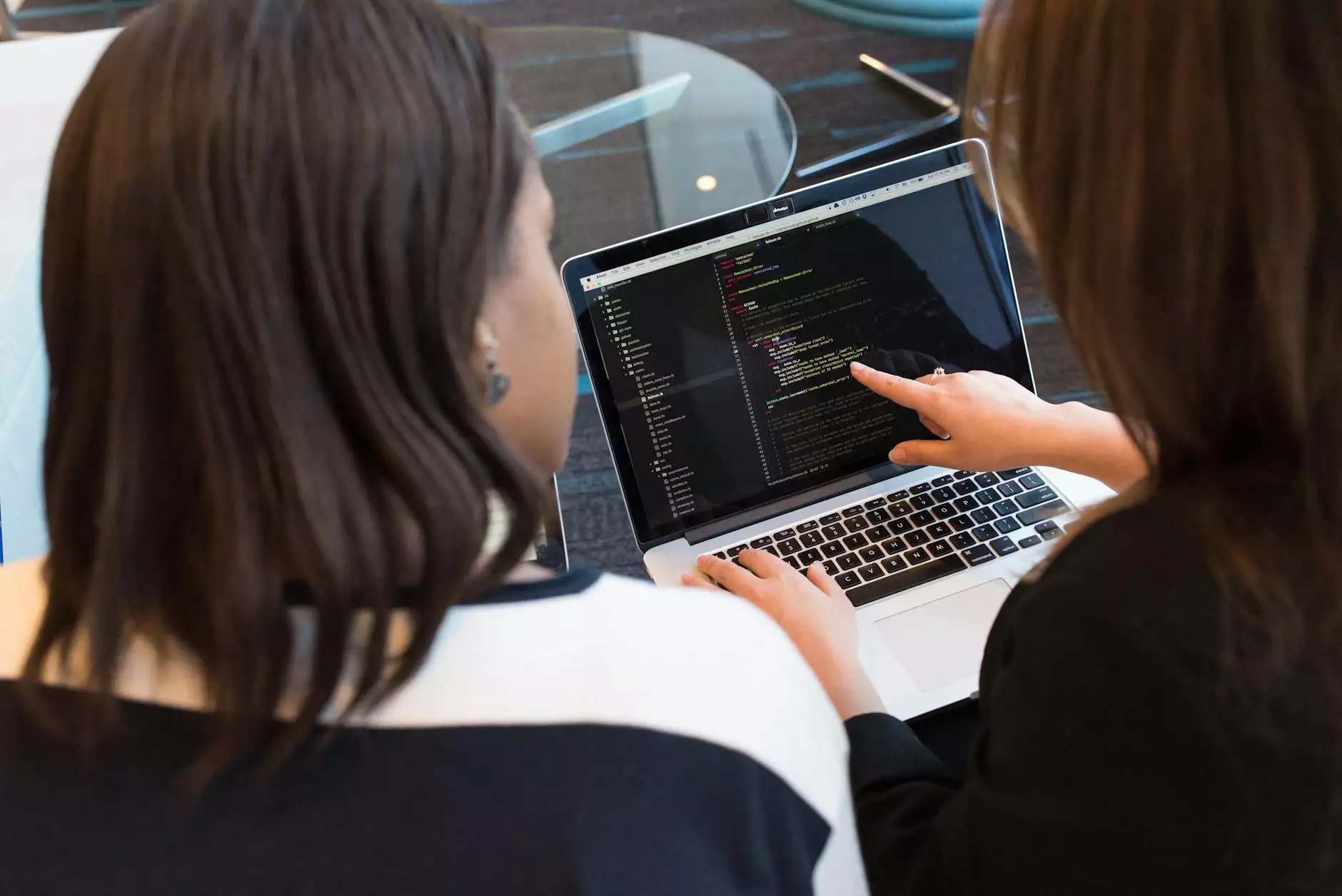
In today's competitive landscape, data labeling machine learning has become a cornerstone for businesses looking to harness the power of artificial intelligence (AI) and machine learning (ML). By assigning meaningful labels to data points, organizations can train more accurate models, leading to improved automation and decision-making processes. In this comprehensive article, we delve into the nuances of data annotation, explore its importance in business, and discuss how Keylabs.ai positions itself as a leader in the domain with its state-of-the-art data annotation tools and platforms.
Understanding Data Labeling in Machine Learning
Data labeling, also referred to as data annotation, is the process of categorizing data to make it machine-readable. This is a fundamental step in training machine learning models, where data sets require accurate labeling to ensure high performance.
What is Data Labeling?
Data labeling involves tagging raw data, whether it be images, text, videos, or audio, with labels that provide context. For example:
- Images: Marking specific objects within an image for object detection models.
- Text: Categorizing sentiment in reviews for natural language processing (NLP) tasks.
- Audio: Transcribing spoken words into text for voice recognition systems.
The Importance of Data Labeling in Machine Learning
The effectiveness of a machine learning model heavily relies on the quality and quantity of labeled data. Poorly labeled data can lead to inaccurate predictions and unsupported business decisions. Thus, the significance of data labeling machine learning cannot be overstated:
- Enhanced Model Accuracy: High-quality labeled datasets lead to superior model performance.
- Informed Decision Making: Businesses can leverage insights gained from accurately trained models to forecast trends and make strategic decisions.
- Improved HR and Operations: Automation of processes through machine learning reduces manual effort and error rates.
Keylabs.ai: Pioneering Data Annotation Tools and Platforms
As a leader in the field, Keylabs.ai offers robust data annotation tools designed to facilitate efficient and accurate data labeling. Our platforms streamline the annotation process, ensuring businesses can focus on their core activities while leveraging the power of AI.
The Key Features of Keylabs.ai’s Data Annotation Tools
Keylabs.ai boasts a variety of features that enhance data labeling for machine learning:
- AI-Assisted Annotation: Our platform employs AI algorithms to automate parts of the annotation process, improving efficiency.
- User-Friendly Interface: With an intuitive design, users can easily navigate the annotation workflow.
- Quality Control Mechanisms: We implement various quality checks to ensure the accuracy and relevance of labeled data.
- Scalability: Our tools can handle projects of any scale, accommodating businesses as they grow.
- Multi-Modal Support: Support for diverse data types including image, video, audio, and text allows businesses to train models on a wide range of applications.
Applications of Data Labeling in Various Industries
The implications of data labeling machine learning reach various sectors, each benefiting uniquely from accurate data annotation:
Healthcare
In healthcare, accurate data labeling is crucial in diagnostics and patient care. For example:
- Labeling medical imagery for automatic tumor detection.
- Classifying patient records for personalized treatment plans.
Finance
The finance industry utilizes data labeling for fraud detection systems and algorithmic trading. By analyzing transaction patterns through labeled datasets, financial institutions can:
- Detect anomalies in spending.
- Predict stock trends based on historical data.
Retail
In retail, businesses employ annotated data to enhance customer experience and optimize inventory management through:
- Analyzing customer sentiment through labeled reviews.
- Employing image recognition for inventory tracking and management.
The Future of Data Labeling and Machine Learning
The future of data labeling machine learning is characterized by advancements in technology and the growing necessity for automation. As businesses increasingly adopt machine learning, the demand for precise data labeling continues to rise. Here are some trends that indicate where the industry is heading:
1. Increased Demand for Automated Solutions
While the manual labeling process has its place, the shift towards automated and semi-automated solutions is becoming apparent. This transformation is crucial for scaling up machine learning projects without compromising quality.
2. Growth in Unlabeled Data Utilization
Many organizations are sitting on vast amounts of unlabeled data. The future will likely see more sophisticated methods of automatically extracting valuable information from these data sources.
3. Expanding Role of AI in Annotation
AI will play an increasingly significant role in streamlining annotation processes, where machine learning techniques themselves assist in labeling data, reducing human workload while increasing accuracy.
Conclusion
Data labeling is a pivotal process within machine learning that directly influences the success of businesses leveraging technology to enhance operational efficiency and decision-making. Through advancements in data annotation tools and platforms, particularly those offered by Keylabs.ai, organizations can better navigate this complex landscape and realize the full potential of their data assets.
Embracing robust data labeling machine learning processes not only positions businesses at the forefront of their industries, but also equips them to tackle future challenges head-on. As we move forward into a data-driven age, the importance of accurate labeling will only continue to grow.